CRC 183
Summer School
Machine Learning
in Condensed Matter Physics
July 16 to 18, 2021
ONLINE EDITION
Machine Learning in Condensed Matter Physics
• learning phases of matter
• neural quantum states
• machine learning and tensor networks
INTRO
The field of machine learning and artificial intelligence has flourished over the past years, fueled by a new generation of algorithms, ever growing resources of computational power, and the availability of “big data” in many fields. These technological advances have a strong impact also on the natural sciences, motivating researchers across all fields to explore machine learning techniques as an extension of their numerical toolbox.
This summer school will cover applications of machine learning (ML) approaches in condensed matter physics including their relation to established techniques. In fact, dealing with the high dimensional and complex data that naturally arise in many-body physics seems to call exactly for the proven strengths of ML techniques — pattern recognition and dimensional reduction. The school will illuminate how ML approaches can help us to identify and analyze phase transitions based on microscopic data. Furthermore, we will see how deep learning can help us tame the “curse of dimensionality” that is inherent to quantum many-body systems by representing physical quantum states as artificial neural networks. Finally, we will sound out connections between ML and tensor networks, which have proven their remarkably versatile utility over the past decades in condensed matter physics.
ORGANIZERS: Augustine Kshetrimayum (Freie Universität Berlin) | Daniel Kaplan (Weizmann Institute) | Markus Schmitt (Universität zu Köln) | Sukhbinder Singh (Monash University, Australia)
Contact: crcsschool2021@gmail.com
Video recordings of the presentations:
Invited speakers:
Giuseppe Carleo
(École Polytechnique Fédérale de Lausanne)
Evert van Nieuwenburg
(Niels Bohr Institute, Copenhagen)
Lei Wang
(Chinese Academy of Sciences, Beijing)
Timetable
Friday, July 16
9:00am – 10:30am
Lei Wang (colloquium)
Scientific machine learning with and without data (overview talk)
10:30am – 11:00am
break
11:00am – 12:30am
Giuseppe Carleo
Neural Quantum States I (generalities and ground state properties)
12:30pm – 14:00pm
break
14:00pm – 15:30pm
Evert van Nieuwenburg
Detecting phases and transitions using supervised learning
15:30pm – 16:00pm
break
16:00pm – 17:30pm
Contributed talks
Saturday, July 17
9:00am – 10:30am
Lei Wang
Generative models (mostly normalizing flows)
10:30am – 11:00am
break
11:00am – 12:30pm
Lei Wang
Differentiable programming (for tensor networks and quantum circuits)
12:30pm – 14:00pm
break
14:00pm – 15:30pm
Giuseppe Carleo
Neural Quantum States II (dynamics/circuits and tomography)
15:30pm – 16:00pm
break
16:00pm – 17:30pm
Hands-on session
Jax, NetKet and quantum phase transitions
Sunday, July 18
9:00am – 10:30am
Evert van Nieuwenburg
Detecting phases and transitions using unsupervised learning
10:30am – 10:45am
break
10:45am – 12:15pm
Hands-on session
Jax, NetKet and quantum phase transitions
12:15pm – 12:30pm
break
12:30pm – 13:00pm
Closing session
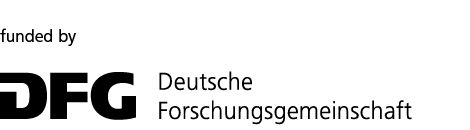
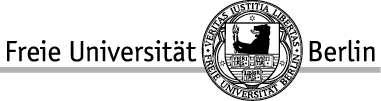

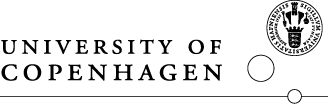
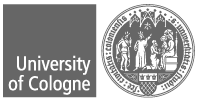